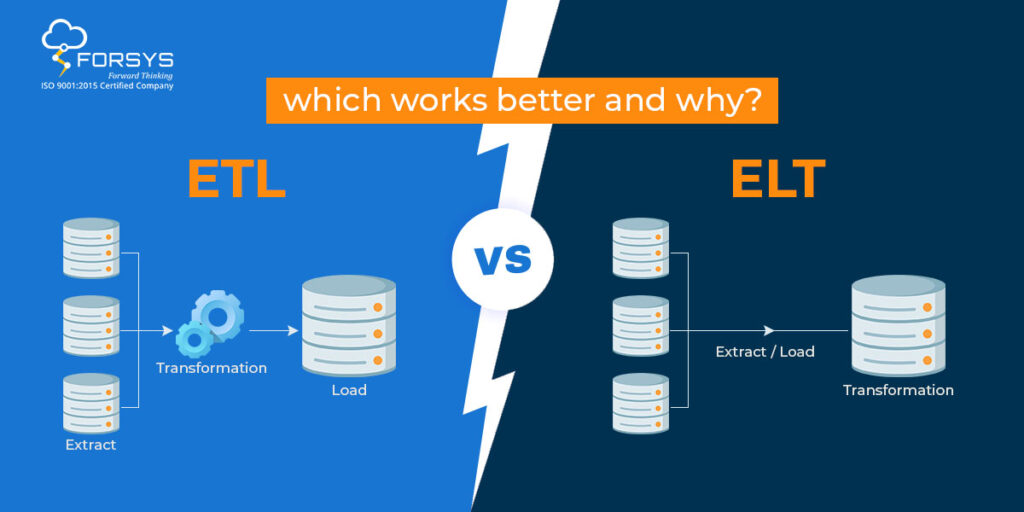
Introduction
Over the last decade, one of the most dramatic outcomes of data explosion has been the massive strain it has put on data warehouse architecture. IDC predicts that by 2024 the overall volume of data created, captured and consumed globally will reach 149 zettabytes every year. And much of this data will be unstructured.
The data explosion is real, the likes we have never really seen before. Nowadays, businesses handle large data volumes of different types from varied sources. And to derive value from them, organizations should be able to sort through them that is achievable when using a data warehouse.
Are you using a data warehouse or planning to build one? To maximize the full potential of an enterprise data warehouse, it is imperative to understand the differences between ETL and ELT, how each of these approaches works and which one is most suitable for new-age businesses.
What is ETL?
ETL stands for extract, transform, load. In this traditional data warehousing and analytics method, data is extracted from one or more data stores, merged/transformed (raw data modified into the required format/type), and then loaded into its destination (i.e., enterprise data warehouse). This has been around for a while and used for data migration.
What is ELT?
ELT signifies extract, load, transform. Unlike ETL, after data extraction, it is first uploaded to the destination where the transformation happens.
What are the key differences between ETL and ELT?
While both the approaches differ, they serve to fulfil the same need, i.e., readying data for analysis to unearth actionable business insights. The comparison of the below-mentioned differences is based on the fundamental features of these approaches.
- ETL leverages a staging server to load the data first and then into the destination database, whereas ELT allows loading the data directly to the targeted database.
- ETL is most suitable for on-premises, relational and structured data, while ELT can support the needs of scalable cloud structured and unstructured data sources. Simply put, ELT is perfect for unstructured data.
- ETL comes with the limitation of handling small or limited data (varying from a few GB to a few TB), while ELT can easily handle large data volumes without any technical hiccups.
- The conventional approach of ETL doesn’t allow it to offer data lake support, whereas serverless event-driven ELT is most suitable for data lakes.
- ETL’s implementation is defined as easy and hassle-free, while ELT’s implementation and maintenance require niche skills.
- ETL has a higher upfront cost and lacks timeliness, whereas ELT’s widespread popularity is due to its ability to deliver a project within a defined time frame and budget.
- A cloud-based ETL needs software installation in a cloud virtual machine, while ELT is all cloud-based and needs no additional hardware.
Is ELT a better option than ETL?
Yes, ELT is considered a better choice. Reason? It is easier to move to a data warehousing structure and support big data initiatives. Since ETL directly feeds conventional data warehouses, loading poor quality data or any data variant that needs significant migration is a strict no-no unless you have a team for writing custom codes to support complex data transformations. In ELT, worrying about the data type isn’t needed as the transformation happens in the targeted database.
Moreover, ETL is outdated as the companies’ shift to the cloud is on the rise. There’s no denying that ETL helped to tackle the limitation of the conventional data centre infrastructure. Having said that, with more businesses moving to the cloud, ELT holds the key to the future of data warehousing as it is agile, requires less maintenance and enables businesses of all sizes to capitalize on the current technologies to unearth insights.
Does Forsys offer data integration and migration services?
Yes, we do. Forsys is a pioneer in data migration with deep domain expertise in leading ERP, SCM, CRM and HCM systems, with pre-built integration templates to empower enterprises to quickly migrate their data and processes and create personalized customer experiences. Forsys’ specialist team uses an agile migration strategy to help enterprises achieve the desired migration volumes needed to digitally transform and bridge the gaps between data, systems, applications and IT processes.
The bottom line
It doesn’t matter if you are working on cloud migration, data warehousing, machine learning or any other data projects, using the data integration and migration approach is the way forward. To harness its full power, ELT is the best choice. Ready to discuss your data migration needs with us or want to know how we helped a global business migrate their massive application portfolio in record time? Connect now!